How Well Did 2001: A Space Odyssey Predict Advancements in AI?
The film “2001: A Space Odyssey” turns 50 this year. In the movie an artificial general intelligence (AGI) named HAL 9000 (Hal) performs many of a spaceship’s functions. Hal explicitly says he is “foolprool and incapable of error,” but later the crew determines he has made an error and decides to take him offline. Hal then starts killing the crew members.
I was recently on the radio discussing, “how well did 2001 predict the future of AI?” While not much in AI was working in 2001 at human or superhuman levels, in 2018 much of Hal’s individual abilities are at these levels. Let’s review Hal’s capabilities and discuss where we are today.
Speech Generation [AI is Human Level]
When the movie was released in 1968, speech synthesis was on the horizon, and “Daisy Bell,” the song sung by Hal in the movie, was synthesized in 1961. The first usable systems were developed in the 1970s (e.g., MUSA), and by the 1990s systems worked well, but sounded mechanical. Using deep learning (WaveNet), today’s systems rival the quality of the human voice.
A fear with this technology is that we will soon be able to generate speech from arbitrary people, so impersonating others using their voices alone is a reality as long as enough data is available. The startup Lyrebird has already developed algorithms with this capability.
Speech Recognition [AI is Near Human Level]
Speech recognition is turning spoken words into written words, which is something Hal uses to have conversations with crew members. Speech recognition research started in the 1950s, but it worked poorly when the movie was released. The first useful systems were released in the 1990s (e.g., Dragon Dictate). Using deep learning, today’s systems have a word error rate of under 5%, which is about the same as humans. However, these tests are done without significant levels of noise, with multiple people speaking at once, or with strongly accented speech. While there is still room for improvement, speech recognition systems rival humans in low-noise environments and will likely work just as well as humans in other environments in the next few years.
Speech recognition is distinct from language understanding, which I discuss below.
Face Recognition [AI is Superhuman]
Hal can recognize the faces of all of the crew members, but could likely recognize far more faces. Face recognition has long been of interest for surveillance and security. Since 2014, systems have rivaled humans in their ability to identify faces. Today’s systems are even better and have surpassed our abilities.
This technology poses major risks to privacy. China is deploying it throughout the nation to track individuals using extensive city-wide camera systems. Megvii’s Face++ was used to arrest 4,000 people in China between 2016-2018. A positive side is that missing people can be found rapidly, and the technology has been used to find kidnapping victims.
Lip Reading [AI is Possibly Superhuman]
In 2001, the crew goes into an area where Hal can see them but cannot listen so that they can discuss turning him off, but unfortunately, Hal can read lips. Using deep learning, today’s AIs are arguably much better than humans. In 2018, DeepMind developed a system that misidentified only 41% of words using video alone, whereas human expert lip readers had an error rate of 93%. It would be prudent to test the efficacy of their method on more datasets to assess its robustness. This technology could be used to aid deaf people or could be used for spying.
https://www.youtube.com/watch?v=0BbYeMyxMy0
Playing Board Games [AI is Superhuman]
Hal was shown to play board games (chess) with the crew. For many years, playing chess at a professional level was a major goal of AI researcher and in the late 1990s IBM’s Deep Blue was able to beat the world’s best. Today’s chess AIs are far more powerful. Soon after IBM’s achievement, researchers argued that chess was likely too easy and that Go was a better target. In 2016, DeepMind created AlphaGo, which beat Lee Sedol, one of the world’s best Go players, and in late 2017 they created AlphaZero, a system capable of defeating AlphaGo and one that can also play chess and shogi at superhuman levels.
Interpreting Emotions [AI is Near Human]
In the movie, Hal could recognize emotional expressions. Arguably, AIs can now classify basic emotional expressions in images as well as humans. Unlike some of the other tasks we have discussed, even humans misclassify emotions fairly regularly. AI’s may be able to perform better than humans since they can extract visual features that humans cannot perceive, enabling them to measure heart rate from video alone.
Reasoning and Planning [Unclear]
Hal can reason about its actions and the actions of others. It is difficult to say how well today’s AIs can do this. AI systems are capable of deduction and can answer questions involving reasoning about short stories, such as those in the bAbI dataset. They can also reason about simple visual environments, such as those in the CLEVR dataset. Given a sufficient model of an environment and an ability to explore that environment, systems can also do extensive planning. That said, no system currently seems to exhibit abilities comparable to the range Hal exhibits, but this ability is harder to benchmark than some of the others we have discussed.
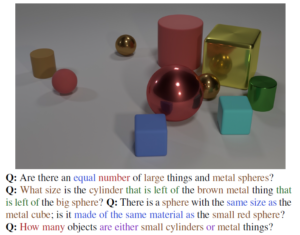
Art Appreciation [Depends]
When Hal finds a crewman drawing, he says, “That’s a very nice rendering, Dave. I think you’ve improved a great deal.” If we assume Hal wasn’t just being polite and actually has this capability, how would it work? Even humans have a difficult time deciding what constitutes good art. One way is to make a system predict if a human art expert would think something was a good work of art, which is something that could very likely be done with a deep neural network that is given enough training data. This would merely be mimicking the opinion of established experts. There are a variety of other methods that could also be attempted. On a related note, AIs can now produce art that is arguably as good as humans. Google’s Magenta project has AIs making artistic images and music. An open question is whether algorithms can go beyond just copying and remixing existing works to create something truly original and unique.
Natural Language Understanding [Unclear]
Hal can communicate with and understand the other crew members, going beyond merely translating their spoken speech into text. Algorithms for parsing sentences work well, but there is significantly more to understanding human speech. Unfortunately, we do not have good metrics for measuring the full range of natural language understanding capabilities that today’s algorithms have compared to humans. To do this ability justice, I’d probably need to break down natural language understanding into many sub-problems and assess how well today’s algorithms work compared to humans. The answer definitely isn’t clear.
Learning Over Time and Autonomously [AI Needs Work]
Deep neural networks learn from massive amounts of labeled data. They learn new things by growing their training datasets. They don’t typically first learn simple things then learn more complex things (curriculum learning). An area of research in my lab is lifelong learning, where we are trying to make AIs that can new skills learn over time in a human-like way, rather than re-training them from scratch when new data is acquired. There is still a gap between state-of-the-art methods for lifelong learning and systems that are trained from scratch every time, but I expect that gap to become small within the next decade. AI still struggles with unsupervised learning and automatically discovering categories in its environment, which is a capability that an AGI should have.
Self-Motivation [AI has a Long Way to Go]
Hal seems to have a lot of self-motivation, but today’s algorithms are largely devoid of these capabilities. How do we endow agents with the ability to teach themselves from unlabeled data? How do we make systems that are motivated to learn interesting things about the world? How do we make systems that are able to set their own objectives in ways that optimize meta-functions of interest to their human users? These are unanswered questions. One of the closest things we have is reinforcement learning, where an AI tries to maximize its reward over time. Making an AI have the right motivations in reinforcement learning to learn the correct behaviors is often quite tricky (reward hacking).
The Ability to Do Multiple Tasks [AI has a Long Way to Go]
Today’s AI algorithms have human-level or superhuman-level capabilities for a wide range of tasks, but these tasks are narrow in scope. This is the distinction between an artificial general intelligence and narrow AI. Hal has a huge range of abilities, but today’s algorithms can typically do only one thing (or maybe a few things) well. Systems struggle to transfer knowledge between tasks. Every task where an AI operates as well as humans has required vast quantities of effort or vast amounts of human-labeled data to achieve that goal.
Final Thoughts
2001 made a lot of predictions that were right as of 2018, but there are still massive gaps between the abilities of today’s AIs and a great generalist system like Hal. One of Hal’s strengths was understanding our intentions and merely our explicit instructions, which is something today’s algorithms cannot do. AGI is being heavily investigated, especially by DeepMind and OpenAI. When I was doing my PhD, talking about AGI was taboo for researchers. It still feels far away, but we are definitely starting to take steps in that direction and narrow AI has approached or exceeded human-level in a number of areas. I’m more worried about automation displacing workers than I am about AI as an existential threat to humanity, but I definitely think AI safety is worth studying.